The 2nd Workshop on Computer Vision in the Wild
@ CVPR 2023, June 19 || 8:45 am - 5:30 pm, PT
Overview
State-of-the-art computer vision systems are trained to predict a fixed set of predetermined object categories. This restricted form of supervision limits their generality and usability since additional labeled data is needed to specify any other visual concepts.
Recent works show that learning from large-scale image-text data is a promising approach to building transferable visual models that can effortlessly adapt to a wide range of downstream computer vision (CV) and multimodal (MM) tasks. For example,
CLIP, ALIGN and Florence for image classification,
ViLD, RegionCLIP, GLIP and OWL-ViT for object detection,
GroupViT,
OpenSeg,
MaskCLIP, X-Decoder, Segment Anything (SAM) and SEEM for segmentation, Multimodal GPT-4, LLaVA and MiniGPT4 for langauge-and-image instruction-following chat assistants.
These vision models with language or interactive interface are naturally open-vocabulary recogntion models, showing superior zero-shot and few-shot adaption performance on various real-world scenarios.
We host this "Computer Vision in the Wild (CVinW)" workshop, aiming to gather academic and industry communities to work on CV and MM problems in real-world scenarios, focusing on the challenge of open-set/domain visual recognition at different granularities and efficient task-level transfer.
To measure the progress of CVinW, we develop new benchmarks for image classification, object detection and segmentation to measure the task-level transfer ablity of various models/methods over diverse real-world datasets, in terms of both prediction accuracy and adaption efficiency. This workshop is a continuation of our
ECCV 2022 CVinW Workshop. For those who are new to this topic, please check out the CVinW Reading List.
-
In this year, our workshop will host two new challenges:
- Segmentation in the Wild (SGinW): Open-set instance/semantic/panoptic segmentation on dozens of semgnetaion datasets in the realistic scenarios.
- Roboflow 100 for Object Detection in the Wild: An augmented version of our ODinW by increasing the datasets to hundreds to cover more diverse application domains.
-
The existing challenges based on the ELEVATER benchmark are also welcoming new submisions:
- Image Classification in the Wild (ICinW)
- Object Detection in the Wild (ODinW)
Keynote Speaker
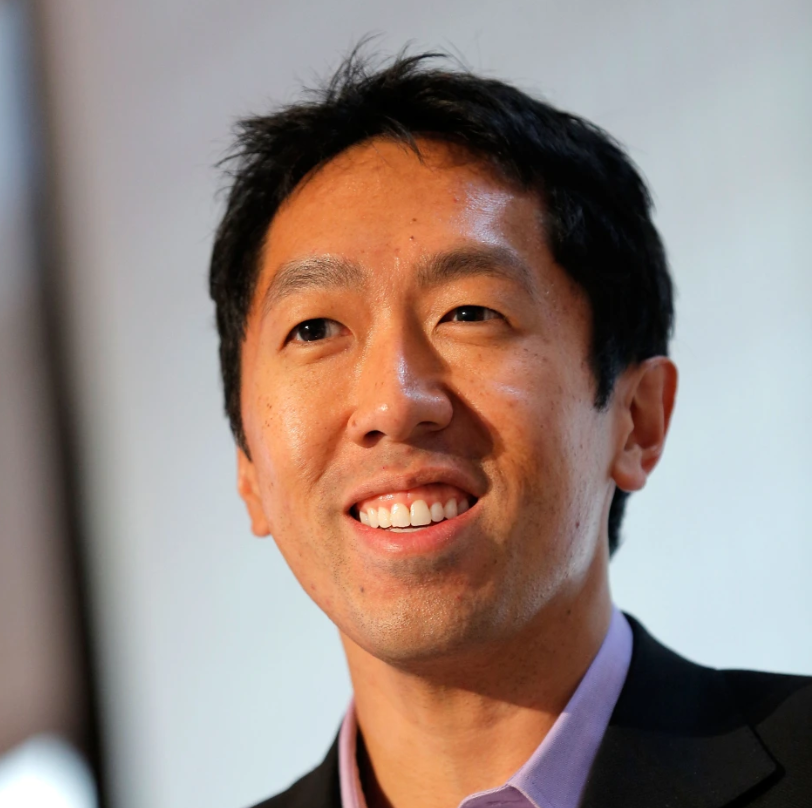
Andrew Ng
Founder of DeepLearning.AI, Landing AI, General Partner at AI Fund, Chairman and Co-Founder of Coursera and an Adjunct Professor at Stanford University.
Invited Speakers/Panelists
Tentative Schedule (June 19th, Monday)
|
![]() |
Welcome
Jianfeng Gao - Microsoft Research |
|
![]() |
KeyNote
Andrew Ng - Landing AI Title: Visual Prompting and the Evolving Workflow of Building Vision Applications Bio [Expand]
Dr. Andrew Ng is the Founder and CEO of Landing AI, whose flagship product is an enterprise AI platform that allows customers to build and deploy AI-powered visual inspection solutions. Dr. Andrew Ng has helped two of the world’s leading technology companies in their “AI transformation”. He was the founding lead of the Google Brain team as well as the Chief Scientist at Baidu, where he led the company’s ~1300 person AI Group and was responsible for driving the company’s global AI strategy and infrastructure.
Dr. Ng is the Chairman and Co-founder of Coursera, the world’s leading MOOC (Massive Open Online Courses) platform, and an Adjunct Professor at Stanford University’s Computer Science Department. His AI courses have had over 7 million enrollments. Dr. Ng has authored or co-authored over 200 research papers in machine learning, robotics, and related fields. In 2013, he was named to the Time 100 list of the most influential persons in the world. He holds degrees from Carnegie Mellon University, MIT, and the University of California, Berkeley.
|
Morning Session On-Site Chair: Xiuye Gu (Google) || Online Coordinator: Haotian Liu (UW Madison) |
||
|
![]() |
Invited Talk
Anelia Angelova Title: From Objects to Scenes: Understanding the Visual World with Vision & Language Models Abstract [Expand]
In this talk we will look at several approaches for leveraging vision and language models for the purposes of object detection in the wild: F-VLM, RO-ViT and FindIt. Furthermore, I will introduce our scaled vision-language models (e.g. PaLI and PaLI-X) which have object detection capabilities. And, lastly, I will present our newest work, MaMMUT, which is a much smaller vision-language model, but capable of many vision-language tasks, including image-text and text-image retrieval, open-vocabulary object detection, video question and answering, video captioning, and others.
Bio [Expand]
Anelia Angelova is a research scientist leading the Vision & Language research team and has previously led the Robot Vision team at Google Research (She is currently in Google DeepMind). Her research focuses on multimodal vision and language models, semantic scene understanding, video understanding, 3D scene understanding and robotics. Anelia received her MS and PhD degrees in Computer Science from California Institute of Technology.
|
|
Spotlight Paper Presentations
|
|
|
Challenge Summary   
|
Challenge Summary
Jacob Solawetz (Roboflow): Roboflow 100 for Object Detection in the Wild Xueyan Zou (UW Madison): Segmentation in the Wild (SGinW) Jiarui Xu (UCSD): Open-Vocabulary Panoptic Segmentation with Text-to-Image Diffusion Models (ODISE) Jiannan Wu (HKU): Universal instance perception as object discovery and retrieval (UNINEXT) |
|
![]() |
Invited Talk
Jiasen Lu - Allen Institute for AI Title: Adaptation or training from scratch? Some preliminary thoughts and experiments towards Multimodal LLMs Bio [Expand]
Jiasen Lu is a Research Scientist at Allen Institute for AI. He obtained his Ph.D. at Georgia Tech, advised by Prof. Devi Parikh. His research is in computer vision, focusing on the intersection between vision and language, and embodiments. He has published at major computer vision (CVPR, ICCV, ECCV), machine learning (NeurIPS, ICLR), and robotics (CORL) conferences and is a co-organizer of the first and second VQA workshops at CVPR.
|
|
![]() |
Invited Talk
Katerina Fragkiadaki - Carnegie Mellon University Title: Open-world 2D and 3D detection, tracking and test-time-adaptation with foundational models Abstract [Expand]
We will first discuss architectures for image and 3D point cloud object open-world detection and referential grounding. Next, we will discuss a general purpose open-world multi-object tracker and segmenter, by re-building previous successful tracking-by-detection methods using a combination of neural modules modern from large scale pretrained discriminative models. Last, we will discuss test time finetuning of large scale pretrained image classifiers using feedback from large scale pretrained generative models. The classifier's parameters are updated at test time to maximize the image likelihood under an image diffusion model that conditions on the inferred classifier label.
Bio [Expand]
Katerina Fragkiadaki is an Assistant Professor in the Machine Learning Department in Carnegie Mellon University. She received her undergraduate diploma from Electrical and Computer Engineering in the National Technical University of Athens. She received her Ph.D. from University of Pennsylvania and was a postdoctoral fellow in UC Berkeley and Google research after that. Her work focuses on combining forms of common sense reasoning, such as spatial understanding and 3D scene understanding, with deep visuomotor learning. The goal of her work is to enable few-shot learning and continual learning for perception, action and language grounding. Her group develops methods for computer vision for mobile agents, 2D and 3D visual parsing, 2D-to-3D perception, vision-language grounding, learning of object dynamics, navigation and manipulation policies. Pioneering innovations of her group’s research include 2D-to-3D geometry-aware neural networks for 3D understanding from 2D video streams, analogy-forming networks for memory-augmented few-shot visual parsing, and language-grounding in 2D and 3D scenes with bottom-up and top-down attention. Her work has been awarded with a best Ph.D. thesis award, an NSF CAREER award, AFOSR Young Investigator award, a DARPA Young Investigator award, Google, TRI, Amazon, UPMC and Sony faculty research awards.
|
|
Lunch Break
|
|
Afternoon Session On-Site Chair: Chunyuan Li (Microsoft) || Online Coordinator: Haotian Zhang (Apple) |
||
|
![]() |
Invited Talk
Kristen Grauman |
|
![]() |
Invited Talk
Ziwei Liu - Nanyang Technological University Title: Towards Building a Practical AI Assistant Abstract [Expand]
The development of practical AI assistants holds tremendous potential for enhancing human-computer interaction by enabling intelligent systems to perceive and understand the visual world. This presentation focuses on the progress made and challenges faced in building such assistants that seamlessly integrate computer vision, natural language processing, and cognitive capabilities. We will delve into our recent research advancements in three key areas: (1) Structured understanding of the world starting from scene relationships, (2) Transition from language models to language assistants, and (3) Transition from multimodal models to multimodal assistants. Through detailed explanations and insights, we aim to shed light on the latest developments in these areas. In conclusion, this talk highlights the exciting advancements in building practical AI assistants that can effectively interpret and interact with the visual world.
Bio [Expand]
Prof. Ziwei Liu is currently a Nanyang Assistant Professor at Nanyang Technological University, Singapore. His research revolves around computer vision, machine learning and computer graphics. He has published extensively on top-tier conferences and journals in relevant fields, including CVPR, ICCV, ECCV, NeurIPS, ICLR, SIGGRAPH, TPAMI, TOG and Nature - Machine Intelligence. He is the recipient of Microsoft Young Fellowship, Hong Kong PhD Fellowship, ICCV Young Researcher Award, HKSTP Best Paper Award and WAIC Yunfan Award. He serves as an Area Chair of CVPR, ICCV, NeurIPS and ICLR, as well as an Associate Editor of IJCV.
|
|
Poster Session (Afternoon Break)
|
Poster boards: #135-#142, WEST exhall A
|
|
![]() |
Invited Talk
Yinfei Yang - Apple AI/ML Title: Image Representation Learning with Grounded Text Abstract [Expand]
The community widely adopts the approach of learning image representations from noisy text supervision. In this study, we introduce a novel method called STAIR, which leverages noisy text supervision to generate a sparse image representation. Our model encodes both image and text inputs into sparse embeddings within a token space, employing a multi-stage training strategy to ensure meaningful token representations. By comparing STAIR with the CLIP model, we demonstrate that STAIR achieves significantly better performance on image-text retrieval tasks. Through quantitative and qualitative analysis, we illustrate that our sparse embedding is more interpretable for humans compared to dense embeddings. Additionally, we propose another method that involves extracting entity information from the noisy text. We utilize this extracted information as labels for the associated images, creating a new dataset with noisy entity annotations. By training a multi-task model on this dataset, we achieve superior performance on image retrieval benchmarks. Moreover, this model exhibits compatibility with image zero-shot learning and linear probing classification benchmarks. The resulting model is named MOFI, which stands for Manifold OF Images.
Bio [Expand]
Yinfei Yang is a research scientist manager at Apple AI/ML working on general vision and language intelligence. Previously he was a staff research scientist at Google research working on various NLP and Computer Vision problems. Before Google, He worked at Redfin and Amazon as research engineers for machine learning and computer vision problems. Prior to that, He was a graduate student in computer science at UPenn. He received his master’s in computer science. His research focuses on image and text representation learning for retrieval and transferring tasks. He is generally interested in problems in Computer Vision, Natural Language Processing, or combined.
|
|
![]() |
Invited Talk
Justin Johnson |
Panel On-Site Chair: Janwei Yang (Microsoft) || Online Coordinator: Haotian Zhang (Apple) |
||
|
![]() ![]() ![]() ![]() ![]() ![]() |
Panel Discussion
Boqing Gong, Bryan A. Plummer, Katerina Fragkiadaki, Dhruv Batra, Jacob Solawetz, Lei Zhang |
Accepted Papers
-
The following papers were accepted to the CV in the Wild Workshop (Poster boards: #135-#142):
- SITA: Single Image Test-time Adaptation Ansh Khurana (Stanford University)*; Sujoy Paul (Google Research); Piyush Rai (IIT Kanpur); Soma Biswas (Indian Institute of Science, Bangalore); Gaurav Aggarwal (Google)
- Perceptual Grouping in Contrastive Vision-Language Models Kanchana N Ranasinghe (Stony Brook University)*; Brandon S McKinzie (Apple); Sachin Ravi (Princeton University); Yinfei Yang (Apple); Alexander Toshev (Apple); Jonathon Shlens (Google)
- ViperGPT: Visual Inference via Python Execution for Reasoning Dídac Surís (Columbia University); Sachit Menon (Columbia University)*; Carl Vondrick (Columbia University)
- From Coarse to Fine-grained Concept based Discrimination for Phrase Detection Maan Qraitem (Boston University)*; Bryan Plummer (Boston University)
- A Robust Likelihood Model for Novelty Detection Ranya Almohsen (West Virginia University )*; Shivang A Patel (West Virginia University); Donald adjeroh (West Virginia University); Gianfranco Doretto (West Virginia University)
- ESS: Learning Event-based Semantic Segmentation from Still Images Zhaoning Sun (ETH Zürich); Nico Messikommer (University of Zurich & ETH Zurich)*; Daniel Gehrig (University of Zurich & ETH Zurich); Davide Scaramuzza (University of Zurich & ETH Zurich, Switzerland)
- FixPNet: Attention Guided Fixation Map Prediction With Explicit Image Priors Rakesh Radarapu (Samsung R & D)*; Sudha Velusamy (Samsung India); Anandavardhan Hegde (Samsung R & D); Narayan Kothari (Samsung R&D Institute, Bangalore, India)
- MAtch, eXpand and Improve: Unsupervised Finetuning for Zero-Shot Action Recognition with Language Knowledge Wei Lin (Graz University of Technology)*; Leonid Karlinsky (IBM-Research); Nina Shvetsova (Goethe University Frankfurt); Horst Possegger (Graz University of Technology); Mateusz Kozinski (ICG TUGRAZ); Rameswar Panda (MIT-IBM Watson AI Lab); Rogerio Feris (MIT-IBM Watson AI Lab, IBM Research); Hilde Kuehne (Goethe University Frankfurt); Horst Bischof (Graz University of Technology)
- ImbaGCD: Imbalanced Generalized Category Discovery Ziyun Li (Hasso Plattner Institute)*; Ben Dai (The Chinese University of Hong Kong); Furkan F Simsek (Hasso Plattner Institute); Meinel Christoph (Hasso Plattner Institute, Potsdam Germany); Haojin Yang (Hasso-Plattner-Institut für Digital Engineering gGmbH)
- Transferring Knowledge for Food Image Segmentation using Transformers and Convolutions Grant Sinha (University of Waterloo)*; Krish Parmar (University of Waterloo); Hilda Azimi (NRC); Chi-en A Tai (University of Waterloo); Yuhao Chen (University of Waterloo); Alexander Wong (University of Waterloo); PENGCHENG XI (National Research Council Canada)
Call for Papers
Topics of interest include but are not limited to:
- Open-set visual recognition methods, including classification, object detection, segmentation in images and videos
- Zero/Few-shot text-to-image generation/editing; Open-domain visual QA & image captioning & Multimodal instruction-following chatbot
- Unified neural networks architectures and training objectives over different CV & MM tasks
- Large-scale pre-training, with images/videos only, image/video-text pairs, and external knoweldge
- Efficient large visual model adaptation methods, measured by #training samples (zero-shot and few-shot), #trainable parameters, throughput, training cost
- New metrics / benchmarks / datasets to evaluate task-level transfer and open-set visual recognition
We accept abstract submissions to our workshop. All submissions shall have maximally 8 pages (excluding references) following the CVPR 2023 author guidelines. All submissions will be reviewed by the Program Committee on the basis of technical quality, relevance to scope of the conference, originality, significance, and clarity. Ther review process is double-blind, and the accepted papers are NOT archived in CVPR proceeding.
Workshop Paper Submission Portal:
[CMT]
Computer Vision in the Wild Challenges
The two new challenges are developed:
|
|
|
|
![]() |
|
|
|
![]() |
![]() |
|
|
|
![]() |
![]() |
-
The two existing challenges associated with this workshop: "Image Classification in the Wild" (ICinW) and "Object Detection in the Wild" (ODinW). We summarize their evaluation datasets and metrics in the table below.
- For the academic track, pre-training data is limited: (1) ICinW: ImageNet21K (Removing ImageNet1K), CC3M+CC12M, YFCC15M; (2) ODinW: Objects365; (3) SGinW: COCO, RefCOCO-g.
- For the industry track, there is no limitation on pre-training data and model size. Teams are required to disclose meta info of model and data if extra data is used. Here are some publicly available image-text datasets: (1) FLAVA Public Multimodal Datasets (PMD) corpus with 70M pairs; (2) LAION with 400M or 5B pairs.
|
|
|
|
![]() |
|
|
|
![]() |
![]() |
|
|
|
![]() |
![]() |
Please see the submission pages for detailed requirements in each Challenge -> Track -> Phase. More information about the challenge benchmark is released: [Benchmark] [Document] [Data Download]. Please reach out if you have any issue in submissions.
Dates
Feb, 2023
Competition starts, testing phase begins
June 2nd, 2023
Competition ends (challenge paper submission)
April 28st, 2023
Workshop paper submission deadline
May 19th, 2023
Workshop paper acceptance decision to authors
June 2nd, 2023
Camera-ready submission deadline
Workshop Organizers
Challenge Organizers and Participants
Program Committee
Yu Sun (UC Berkeley)
Dequan Wang (SJTU)
Jiarui Xu (UCSD)
Feng Li (HKUST)
Liangyu Chen (NTU)
Rishi Madhok (Microsoft)
Shilong Liu (Tsinghua Univ.)
Yangming Wen (UC Davis)
Haotian Liu (UW Madison)
Yiwei Zhang (UW Madison)
Gaoang Wang (ZJU)
Liangchen Song (Buffalo)
Haorui Ji (Univ. Washington)
Xin Wen (HKU)
Nan Pu (Univ. of Trento)
Sagar Vaze (Oxford Univ.)
Xiaodan Hu (UIUC)
Dongze Lian (NUS)
Jianwei Yang (MSR)
Xueyan Zou (UW Madison)
Hao Zhang (HKUST)
Yiwu Zhong (UW Madison)
Mengde Xu (HUST)
Yibing Wei (UW Madison)
Workshop and Challenge Questions?
Reach out: https://github.com/Computer-Vision-in-the-Wild/cvpr-2023
Workshop Organizing Team